Portfolio Managers are rushing to bring AI into their investment process, collectively acknowledging the invaluable benefits it adds to their decision-making, from managing risk and diversification more effectively, to achieving a superior risk-adjusted performance.
During our latest live event, Axel Maier, CFA (Partner at MDOTM Ltd), Lorenzo Malanga (Vice President Of Research) and Joana Fernandes (Client Solutions Manager), moderated by Andrea Sala from MDOTM Ltd, discussed how AI, especially Sphere, can help multi-asset portfolio managers combine their investment views with AI’s global outlook, create customised portfolios based on their specific investment objectives and enhance them.
The following are the key takeaways of the discussion.
Why Portfolio Managers Need AI To Manage Market Complexity
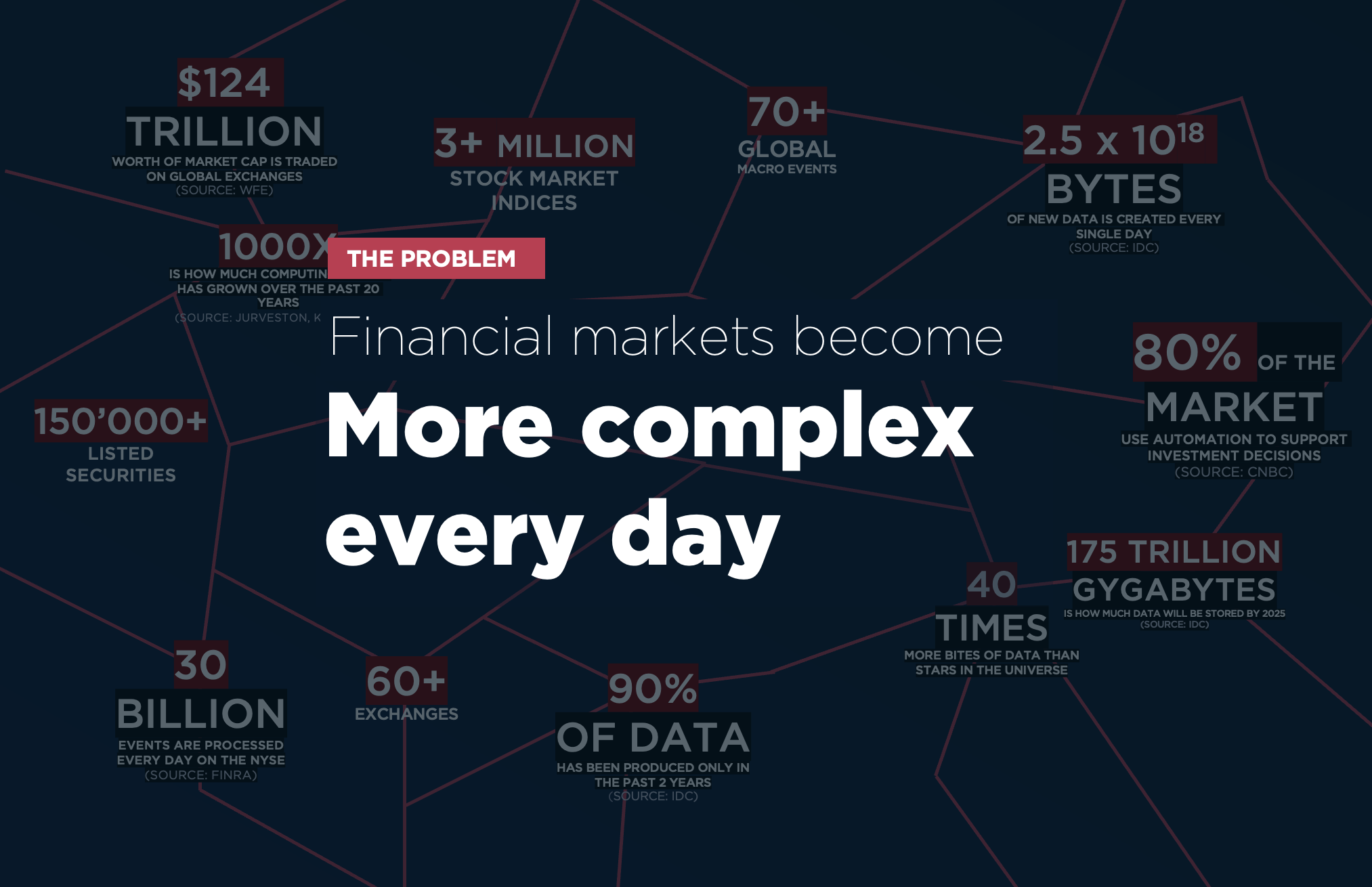
The rapid growth in data volume and complexity over the last few decades has had a profound impact on multiple industries, and financial markets are no exception. As technology has evolved and become more sophisticated, the amount of data available to market participants has increased exponentially, making it more difficult to identify meaningful patterns and insights. This explosion of data has made financial markets more complex, presenting significant challenges for institutional investors. In this context, the ability to analyse large and diverse datasets has become a crucial element in making more informed decisions and gaining an edge over competitors.
This is where AI can offer valuable assistance. On a general level, AI in data analysis is already used across different industries and it brings huge benefits. AI technologies, in a complex and rapidly changing market, have become an indispensable tool for any professional, but especially institutional investors, looking to maintain a competitive edge.
Alpha Generation: The True Value-Add of AI in the Investment Process

Artificial Intelligence applied to investment management is providing new opportunities which extend beyond cost reduction and efficient operations. By analysing millions of datapoints every day, AI can help investors anticipate and forecast future market trends with a higher degree of accuracy (what we call Forecasting Alpha), while also enabling them to adhere to a well-defined strategy to systematically achieve an optimal portfolio construction (what we call Portfolio Construction Alpha).
The Methodology Used By Sphere
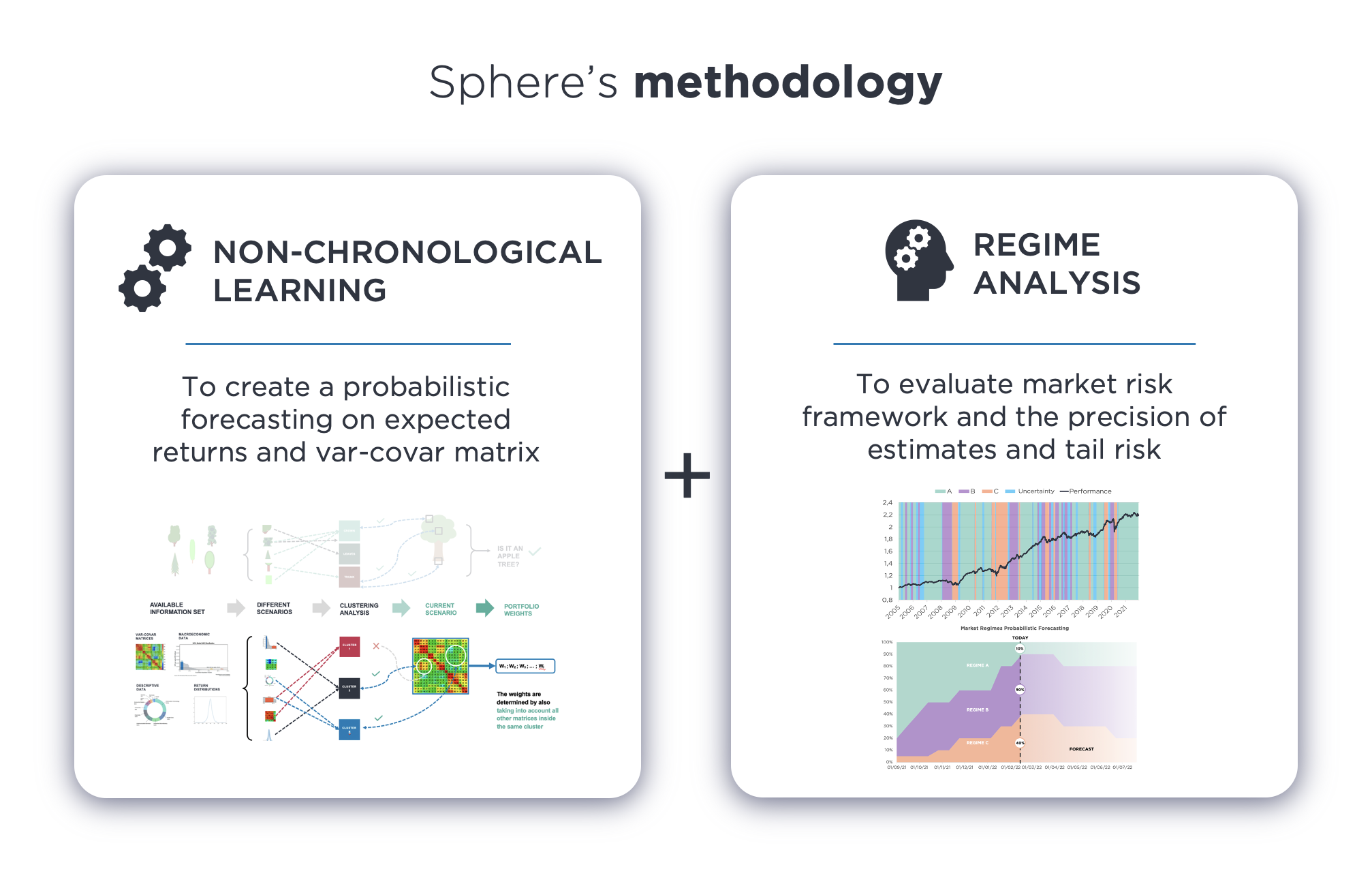
The Human decision process is driven by two key components:
- Experience: we combine past events in a non-linear way through our imagination;
- Data Evaluation: we look at data to have the “best” possible understanding of the status quo.
When it comes down to making an investment decision, an experienced portfolio manager usually follows the same path: to make an educated guess on what could happen in the future, the Portfolio Manager would start by remembering different past market phases, past market dynamics, past black swan events and then would look at today’s context, gathering as much information as possible to combine it in a “non-linear” way. AI also utilises Experience and Data Evaluation, but differing from humans in how it does it: AI does it through leveraging data. This is why such an approach pairs up very well with a more traditional/fundamental one. Humans have an edge when information is scarce but AI, on the contrary, has an edge when information is abundant and when it is a matter of connecting and analysing thousands of data points altogether.
MDOTM leverages its proprietary AI methodology to provide actionable investment insights based on a joint evaluation of the following components:
- Experience, which is gained through a methodology that we called Non-Chronological Learning: a clustering analysis of past market dynamics and events.
- Data Evaluation, which happens through a methodology that performs Regime Analysis: a derivation of HMM (Hidden Markov Model) that allows us to assess in which type of risk framework we are in – see below.
How To Construct and Enhance Portfolios with AI
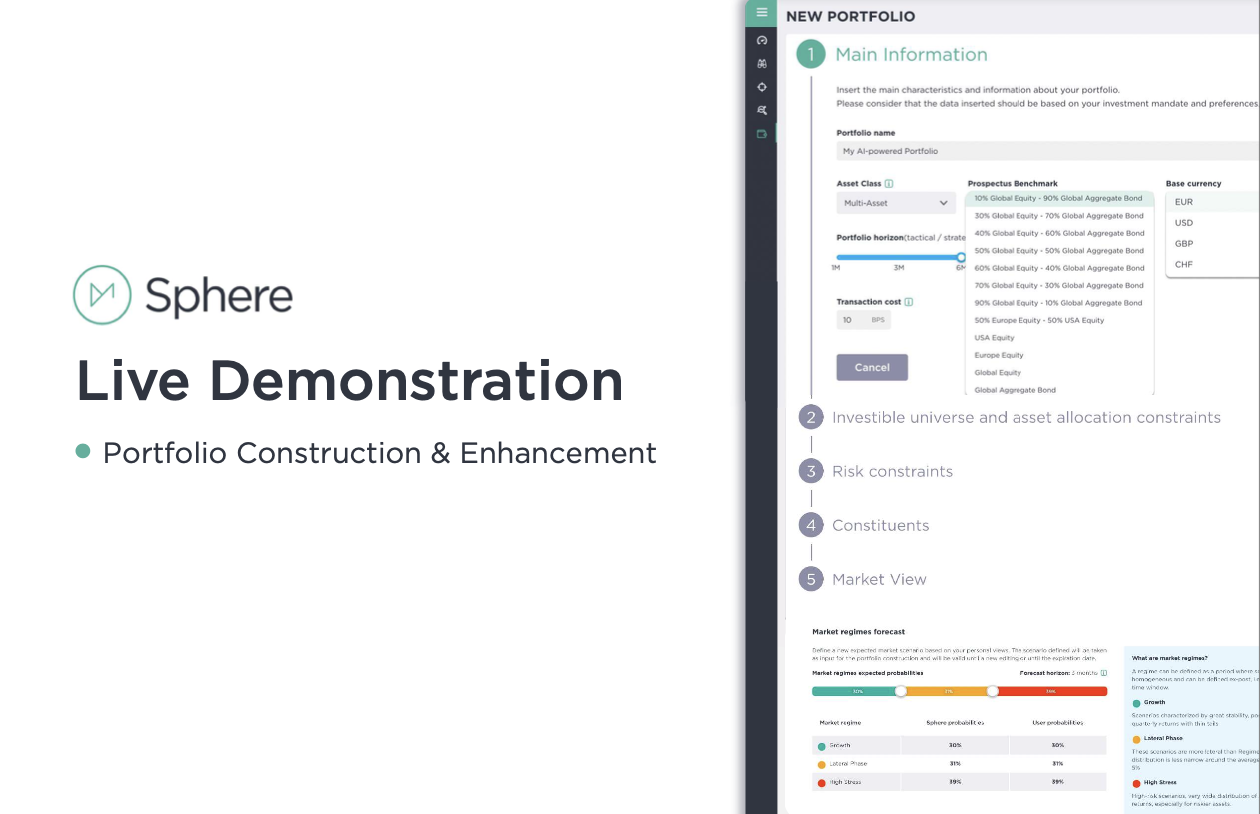
With Sphere, investment professionals can design their portfolios in every aspect, leveraging our AI to easily integrate constraints, complex investment objectives, and custom views. From base currency to asset allocation constraints and more, the customisation at scale offered by Sphere makes it a solution that can be autonomously integrated and enrich any professional’s investment process. Sphere provides relevant information so decision-makers can actively interact with it and proceed with their investment decisions. Our platform doesn’t exclude the manager’s point of view, but empowers it with investment choices assisted by AI’s inputs for a constant monitoring of risks, a dynamic analysis of market regimes, diversification between asset classes, and much more. Through assisted decision-making, Sphere ensures that investment professionals always remain at the centre of any investment decision.
Combining Your Views With an AI-Driven Market Outlook
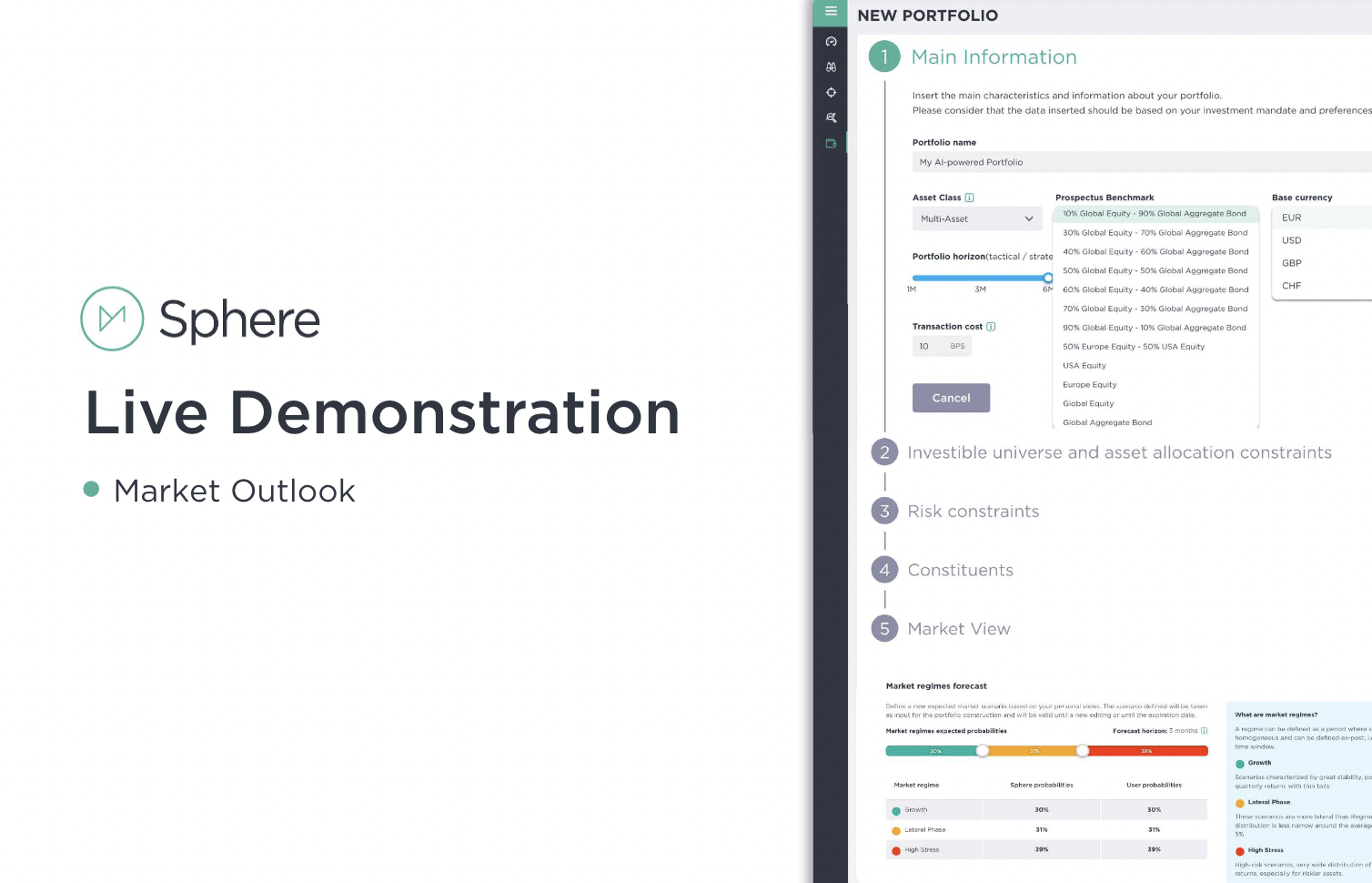
Everyday financial markets are in a defined regime characterised by the existing dynamics, conditions, and behaviour of different components and variables. In today’s context of growing complexity, it is crucial to be "regime-aware” when making investment decisions as it allows better positioning of the portfolio’s risk. Sphere's Market Regime Analysis is done by using a derivation of Hidden Markov Models (HMM), an unsupervised machine learning technique generally used to estimate forward-looking probabilities and to understand the evolution of complex systems that cannot be directly observed. The HMM bottom-up approach to market segmentation allows identifying market regimes in terms of risk environment and data coherence, adding value compared to mere return/growth measurements.
The 5 Steps For AI Adoption
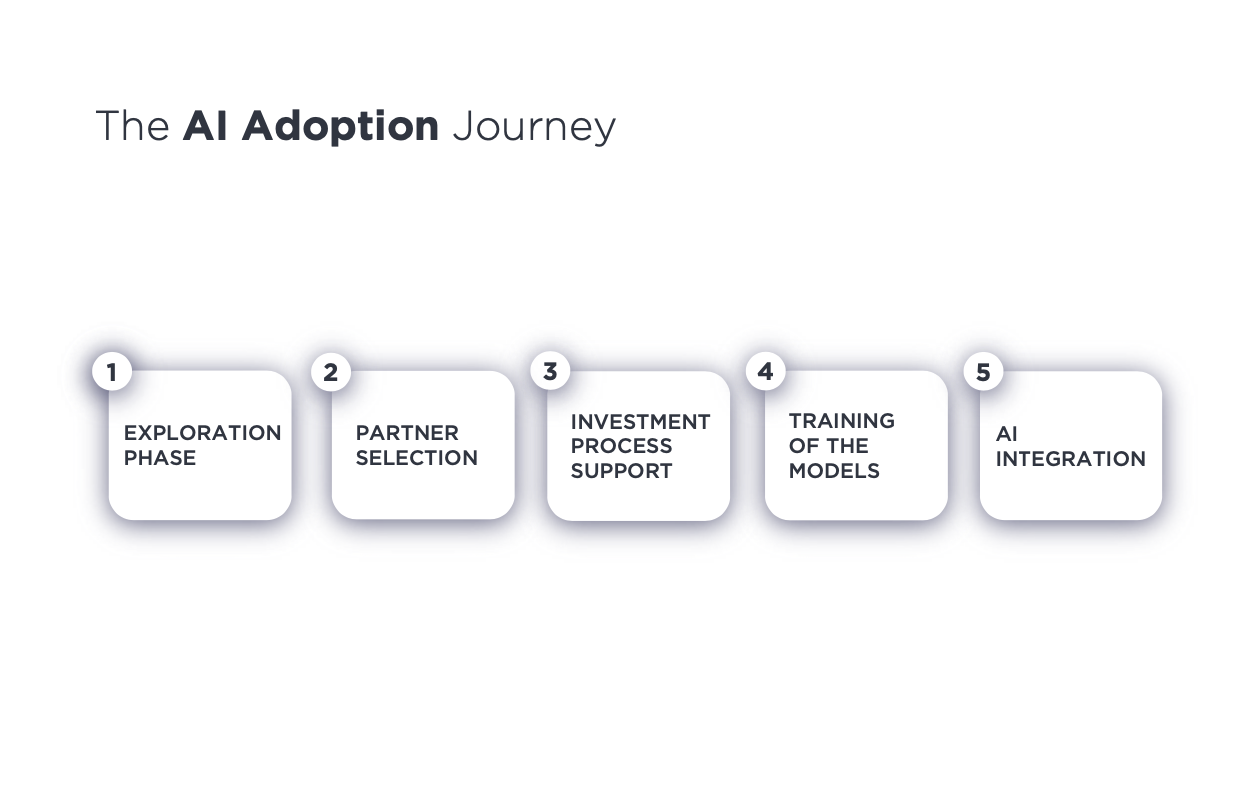
Even though the final output of AI’s Adoption may differ from one institution to another according to each one’s objectives and requirements, there are many common traits that serve as a baseline to assess where your institution is in this journey.
Step #1: Exploration Phase - During the Exploration Phase, financial institution’s decision-makers typically investigate and explore the benefits of AI applied to a specific part of their process, identifying the areas where AI can provide valuable insights and improvements.
Step #2: Partner Selection - As a result of the Exploration Phase, clients typically end up with a range of potential solution providers and then evaluate, based on a specific set of rigid criteria, whether their solution can be applied into their existing framework and how reliable it is.
Step #3: Investment Process Support - At this point, investment professionals need to analyze and break down each step of their existing investment process. This examination helps them identify specific areas where AI can provide valuable support, such as automation, data analysis, or pattern recognition. By pinpointing these opportunities, they can strategically integrate AI to enhance decision-making.
Step #4: Training of the Models - To align AI with investors’ unique requirements, it’s pivotal to train AI models to fit in their investment process precisely. This step involves feeding the models with relevant historical data and refining them to provide accurate insights. Tailoring the models to specific investment objectives and risk tolerance ensures optimal performance.
Step #5: AI Integration - This step involves combining the insights generated by AI models with the expertise and perspectives of your asset management team. This integration allows asset managers to leverage the capabilities of this technology while maintaining human judgment, ultimately enhancing the decision-making process. Of course, after such integration, continuous monitoring and optimization are essential for successful AI adoption. Regularly assess the performance and effectiveness of the integrated system and make adjustments and improvements as needed to maximize the value and benefits derived from AI.
---------
Start your AI Adoption Journey today
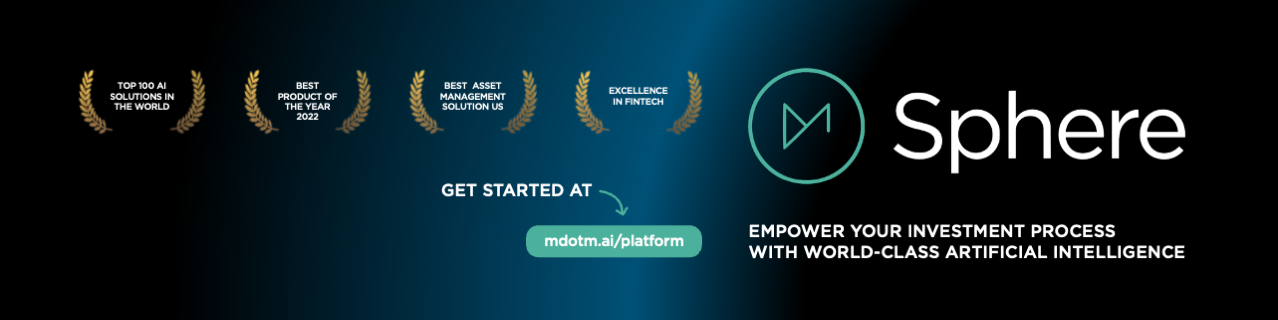