This article explores the benefits of generative AI in investment management. Generative AI is revolutionising the way investment strategies are developed, implemented, and optimised. By harnessing the power of machine learning algorithms, generative AI offers a range of advantages that can significantly enhance decision-making processes and drive better investment outcomes.
‍
Understanding Generative AI
Generative AI is the branch of artificial intelligence that focuses on creating new content, such as images, texts, and sounds, based on patterns learned from existing data. It differs from other AI techniques by enabling machines to generate original, unique outputs rather than simply analysing and categorising existing data. The application of generative AI in investment management opens up new possibilities for uncovering hidden patterns, generating novel investment strategies, and predicting market trends with greater accuracy.
‍
Generative AI comprises algorithms that can create and produce new data samples that resemble the training data it has been exposed to. By capturing the underlying patterns and structures in the data, generative AI can generate new insights, ideas, and solutions.
‍
One popular technique used in generative AI is the generative adversarial network (GAN) framework, which consists of two neural networks: a generator and a discriminator. The generator aims to generate new data samples, while the discriminator tries to differentiate between the generated samples and real data. Through an iterative process, these two networks learn from each other, constantly improving and refining the quality of the generated outputs.
‍
The field of generative AI has witnessed significant advancements in recent years. With the availability of large-scale datasets and improvements in computational power, researchers have been able to develop more sophisticated generative models. These models can generate highly realistic images, text passages, and even music that is indistinguishable from human-created content.
‍
Investment management can benefit from these advancements by leveraging generative AI to gain novel perspectives, develop innovative investment explanations, and generate insights that may not be apparent through traditional analysis. By enabling machine-generated explanations, generative AI can unlock new investment opportunities and lead to more profitable investment decisions.
‍
Generative AI has also found applications in various other fields. In the field of art, generative AI has been used to create stunning and thought-provoking artworks. Artists and designers can use generative AI algorithms to generate new and unique designs, pushing the boundaries of creativity.
‍
In the field of music, generative AI has revolutionised the way music is composed. Musicians and composers can now use generative AI algorithms to create original compositions, exploring new melodies, harmonies, and rhythms that were previously unexplored.
‍
Generative AI has even found applications in healthcare. Medical researchers are using generative AI algorithms to generate synthetic medical images and data, which can be used to train and improve diagnostic models. This has the potential to enhance the accuracy and efficiency of medical diagnoses, leading to better patient outcomes.
‍
Furthermore, generative AI has been used in the gaming industry to create realistic virtual worlds and characters. Game developers can use generative AI algorithms to generate lifelike environments, populated with unique and diverse characters, enhancing the immersive experience for players.
‍
As generative AI continues to advance, its potential applications are only limited by our imagination. From art and music to healthcare and gaming, generative AI is transforming various industries and pushing the boundaries of what is possible.
‍
The Intersection of AI and Investment Management
AI has become an integral part of modern investment strategies. Machine learning algorithms can process vast amounts of data, identify patterns, and make predictions much faster than humans. The combination of AI and investment management allows for data-driven decision-making, enhanced risk management, and increased efficiency in portfolio management.
‍
When it comes to investment management, AI plays a crucial role in providing valuable insights into market trends. By analysing historical market data, financial statements, and macroeconomic indicators, machine learning algorithms can identify patterns and correlations that humans may overlook. This ability to process and analyse large volumes of data in real-time gives investors a competitive edge in understanding market dynamics and making informed investment decisions.
‍
Unlocking Insights with Generative and Analytical AI
‍
In investments, two distinct yet complementary AI branches have emerged: analytical AI and generative AI. To understand their significance in investment decision-making, it's essential to grasp their fundamental differences and how they are leveraged in order to guide investment decisions.
‍
Analytical AI: Uncovering Valuable Insights
Analytical AI is the technology that harnesses Machine Learning techniques to delve into data, uncover hidden insights, identify novel patterns, and establish relationships within datasets. This form of AI operates on principles deeply rooted in statistics and mathematics. Some common methodologies employed by analytical AI include Linear Regression, Neural Networks, Decision Trees, and Random Forests.
‍
Analytical AI scrutinises vast volumes of historical and real-time data, aiming to extract meaningful information. In the financial landscape, the role of analytical AI can be exemplified by MDOTM's investment platform, Sphere. Sphere, with its analytical capabilities, meticulously analyses millions of historical, market, and macro data points. This thorough and advanced analysis unveils hidden signals within the noise of data, so then investment professionals can guide accordingly their investment decisions.
‍
Generative AI: Enhancing Explainability
Generative AI has a completely different architecture than analytical AI. Generative AI models learn the patterns and structure of their input data, and then generate completely new data that has similar characteristics. Generative AI also includes a “layer” that facilitates an ongoing human interaction with the technology to produce novel outputs. Generative AI thrives on the power of creativity and imagination, and it often operates in response to specific prompts or requests provided by humans. Unlike analytical AI, which sifts through existing data to uncover valuable insights, generative AI generates entirely new content based on the input it receives.
‍
For example, consider Midjourney, an AI-powered image generation tool. Users interact with Midjourney by providing textual or visual prompts, such as a description of the image they wish to see or even an existing image as a reference. Generative AI like Midjourney then creates a unique image based solely on the user's input.
‍
Applying Generative and Analytical AI to Investment Decisions
Analytical AI excels at sifting through vast datasets, historical market data and economic indicators to identify trends and correlations that humans might traditionally overlook. It provides investment professionals with data-driven insights, risk assessments, and portfolio optimisation strategies. By automating complex data analysis, it frees up human experts to focus on higher-level strategic decisions.
‍
Generative AI plays an important role in ensuring AI explainability in investments. It accomplishes this by generating comprehensive and clear explanations for the analytical output of AI. When investment professionals or clients receive AI recommendations, generative AI can provide detailed reports that break down the rationale behind each recommendation. These explanations shed light on why certain investment choices are made, helping to understand the technology’s reasoning, and ensuring AI explainability.
‍
The Nexus Between Generative and Analytical AI
‍
In investment management, the relationship between generative and analytical AI emerges as a powerful tool, enhancing the way investment decisions can be made and understood.
‍
Analytical AI, with its proficiency in data analysis, serves as the foundation of this partnership. It's the engine that processes vast datasets, examining historical market data, and economic indicators. Analytical AI has the capacity to uncover intricate trends, correlations, and patterns. It provides investment professionals with invaluable data-driven insights, risk assessments, and strategies for optimising investment portfolios.
‍
Generative AI, on the other hand, plays a role in ensuring transparency and explainability in investment decisions. It works with human interaction and operates in response to specific prompts or queries. This aspect of generative AI is particularly significant when applied to investments, as it bridges the gap between the analytical output of AI and the human understanding of the technology’s reasoning.
‍
The synergy between these two forms of AI is most evident with regards to AI explainability. Investment professionals often seek to understand the rationale behind AI-generated investment inputs. While analytical AI can pinpoint trends and suggest portfolio adjustments, generative AI steps in to provide comprehensive explanations. When an investment decision is made based on analytical AI's insights, generative AI can create detailed reports that explain the reasoning behind each recommendation. These reports serve as a critical link between the intricate analyses performed by analytical AI and the decision-makers involved. They break down complex data into understandable narratives, allowing investment professionals and clients to grasp why particular investment insights have been provided.
‍
For instance, if analytical AI recommends a shift in portfolio allocation, generative AI can produce an explanation outlining the underlying factors, such as recent market developments, economic indicators, or company performance metrics. This interpretation empowers investment professionals to make informed decisions and communicate the rationale behind their choices effectively.
‍
The nexus between generative and analytical AI is reshaping the modern approach towards investment management. Analytical AI's data-driven capabilities are harnessed to uncover hidden insights and optimise portfolios, while generative AI ensures these insights are translated into clear and comprehensible explanations. Together, they empower investment professionals with a data-driven precision and human-centric understanding.
‍
AI Integration: From Research to Maturity
‍
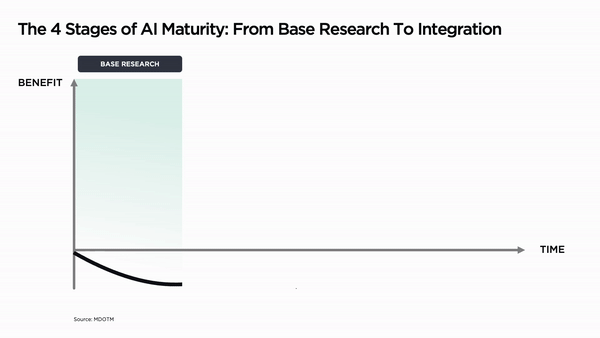
‍
As we've explored earlier, analytical AI excels in data analysis, uncovering insights, and optimising portfolios, while generative AI ensures AI's understandability by providing comprehensive explanations for its decisions. In order to comprehend how these technologies are being integrated into the investment process of numerous financial institutions, we will delve into each stage of our AI maturity model, shedding light on the journey from Base Research up to the actual Integration. To illustrate our research, we will also draw upon Gartner's AI maturity model, which segments companies into five levels of maturity regarding their use of AI.
‍
1. Base Research: Laying the Foundation for AI
The Base Research stage aligns with the initial awareness of AI's potential (Level 1 in Gartner's model). During this phase, organisations recognise the power of AI but have yet to harness it effectively. MDOTM refers to this phase as the Base Research stage. It is where the groundwork is laid, where we invest in understanding the AI landscape, its capabilities and limitations.
‍
2. Applied Research: Experimentation and Learning
As organisations advance to Gartner's Level 2 - Active, they begin to experiment with AI in practical settings, transitioning from mere awareness to active experimentation. This phase corresponds to the Applied Research stage, where AI applications move from ideation to practical applications. When AI is still in its developmental stages, it starts to demonstrate practical value through experimentation and learning.
‍
3. Custom Applications: Tailoring AI to Specific Needs
At Gartner's Model Level 3 - Operational, companies have embraced Machine Learning, deploying it in daily operations. Here, AI is tailored to meet specific requirements, ensuring that solutions align precisely with clients' objectives. Here, the technology begins to deliver more substantial benefits compared to its earlier stages, providing clients with highly customised and tangible value.
‍
4. Integration: AI as an Integral Part of Investments
Finally, at Gartner's Level 4 - Systemic, companies fully integrate AI into their processes and infrastructure. In this stage, the technology becomes an integral part of investment decision-making. It actively shapes investment strategies and provides forward-looking, timely insights.
‍
The seamless interaction between generative and analytical AI, coupled with their progression through the stages of AI maturity, leads to a future where AI-driven investment management is not just a choice but a necessity to have a competitive edge in the financial industry. This approach empowers investors to make informed decisions, optimise portfolios, and thrive in an ever-evolving landscape. As the financial industry continues to evolve, the partnership between generative and analytical AI will play a pivotal role in ensuring AI's maturity, explainability, and effectiveness in investment management.
‍